A research team from the University of Pennsylvania has reported creating a high-resolution dataset by scanning 135 ex vivo human brain tissue specimens using a 7 Tesla MRI, which provides high-resolution imaging at high magnetic field strength. The team also performed segmentation using a deep learning pipeline.
Ex vivo MRI offers advantages over in vivo MRI in that it allows for high-resolution examination of brain structures and enables linking pathological measurements with morphometric measurements. However, automatic segmentation for ex vivo MRI brain mapping has not been sufficiently developed due to reasons such as lack of datasets and heterogeneity in scanning hardware and acquisition protocols.
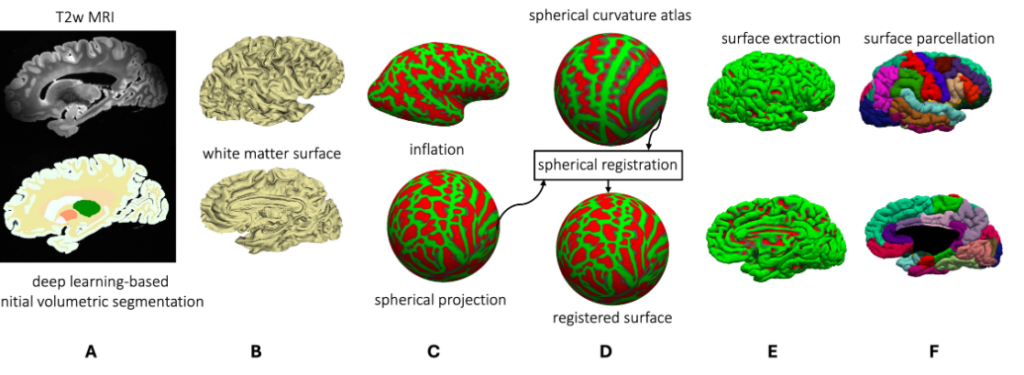
The University of Pennsylvania research team created a high-resolution dataset by scanning 135 ex vivo human brain tissue specimens using a 7 Tesla MRI, which provides high-resolution images at high magnetic field strength. For each brain specimen, they obtained three different MRI sequences: a T2-weighted scan at 300 microns, a KISS scan at 500 microns, and a T2-star F scan at 160 microns. The dataset includes specimens from donors with various primary and secondary diagnoses, including Lewy body dementia, frontotemporal lobar degeneration, and cerebrovascular disease. From the total collection, 82 specimens were specifically grouped in the AD continuum for quantitative analysis.
Similar Posts
They also developed a deep learning pipeline for segmenting cortical regions. Furthermore, they successfully segmented four subcortical structures (caudate, putamen, globus pallidus, and thalamus), white matter hyperintensities, and normal appearing white matter. The team adapted and developed a fast, automated surface-based pipeline to parcellate ultra high-resolution ex vivo brain tissue using the Desikan-Killiany-Tourville (DKT) brain atlas, enabling vertex-wise analysis in template space.
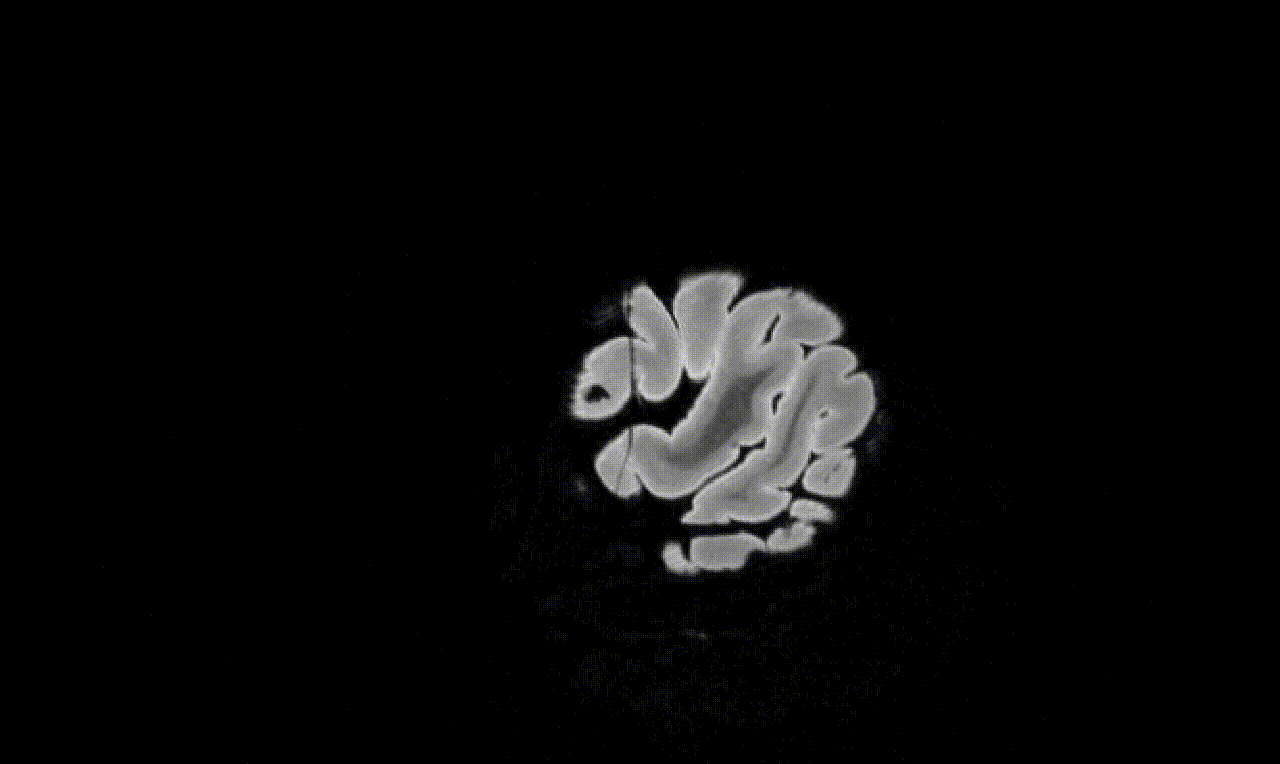
This pipeline demonstrated excellent generalizability across whole brain hemispheres from different specimens and was also able to handle unknown images obtained with different magnetic field strengths and different imaging sequences. Their analysis revealed significant negative correlations between cortical thickness and various pathological indicators, including amyloid-β, Braak staging, CERAD, and medial temporal lobe neuronal loss and tau pathology. The strongest correlations were observed in regions implicated in Alzheimer’s and related dementias, including the entorhinal cortex, parahippocampal, medial orbital frontal, temporal pole, inferior temporal, and perirhinal regions. The code, containerized executables, and processed datasets are publicly available on GitHub.