SpeciesNet is a kind of artificial intelligence (AI) model that has been designed to perform wildlife identification with exceptional accuracy. It has been developed using more than 65 million photos of wild animals taken by camera traps from all over the globe with the aid of WWF (World Wildlife Fund) and other conservation partners. SpeciesNet was released as open-source in 2025, and it can be accessed and utilized for free by anyone. Whether you’re a researcher, wildlife ranger, or student working on a nature project, you can utilize this tool to identify animal pictures more efficiently than ever before.
Dr. Sara Beery, the Homer A. Burnell Career Development Professor at the Massachusetts Institute of Technology (MIT) and a pivotal figure in the development of SpeciesNet, expressed her enthusiasm: “I am incredibly excited to see our SpeciesNet model released!! This represents years of work by the @WildlifeInsights AI team. I truly believe the release of this model will be a transition point for the camera trap community in terms of the open, flexible, scalable use of AI.”
The Camera Trap Problem—And How SpeciesNet Fixes It
Camera traps are autonomous devices that non-invasively capture images of wildlife. They activate when movement is detected, automatically capturing images of potential subjects. However, alongside their numerous advantages, they generate enormous volumes of data. In just a few weeks, a single project can collect tens of thousands of pictures.
Most of these photos don’t contain wildlife—just branches or shadows moved by wind. Only a small percentage of images actually contain animals, and identifying these accurately requires both expertise and time.
These are the two problems that SpeciesNet handles: With a performance rating of 99.4%, it can identify animals in almost all of the frames in which the animals are present, meaning it rarely misses animals that are captured in images. Additionally, the false positive rate is only 1.3%, meaning the model incorrectly identifies an animal in just 1.3% of images that don’t actually contain animals.
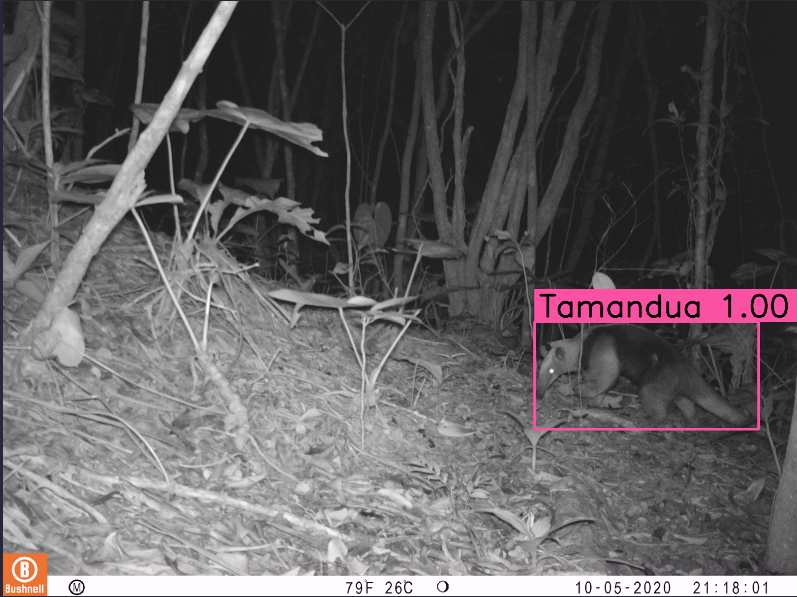
Moreover, when making species-level predictions, the model achieves an accuracy of 94.5%. This demonstrates that in terms of efficiency and precision, no human team could identify subtle differences between similar species like leopards and jaguars, or visually similar birds, with comparable speed and accuracy.
How Does SpeciesNet Work?At its core, SpeciesNet utilizes the EfficientNet V2 M architecture, a convolutional neural network developed by Google that balances accuracy and computational efficiency, making it suitable for large-scale image classification tasks. This is not a hardware chip but rather a software model that can be implemented on various hardware platforms.
Beyond visual data processing, SpeciesNet employs ensemble decision-making, combining outputs from an object detector and an image classifier. Geographic location data also serves as contextual input for the model. For example, a camera trap in Kenya creates an implicit assumption for the AI not to identify species found only in South America. This clever use of geographical prior information helps eliminate common mistakes and enables the model to make well-considered predictions.
The model can recognize animals from over 2,000 categories, including:
- Specific animal species
- Higher-level taxa (e.g., “mammalia,” “felidae”)
- Classification by group size (individual, pair, group)
- “Blank” for empty images (no animals)
- Non-animal classes such as humans or vehicles.
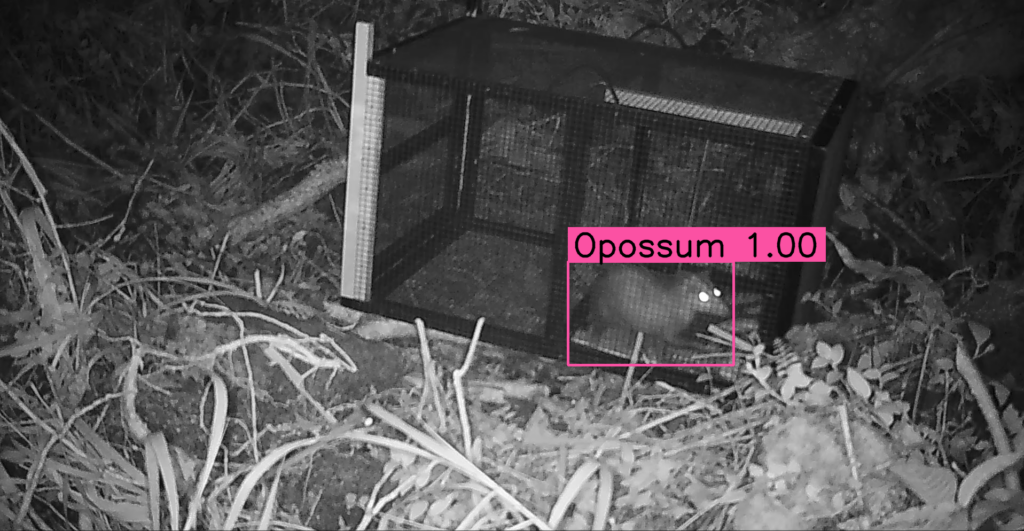
Real-World Impact: SpeciesNet in ActionSpeciesNet is not merely a technological demonstration but an active tool making real-world impact. Since 2019, its applications for wildlife conservation have spread across Asia, Africa, South America, and Australia.
Mike Werner, Head of Sustainability Programs & Innovation at Google, highlighted the significance of SpeciesNet: “We’re also announcing the open source release of SpeciesNet, an AI model designed to identify animal species by analyzing photos from camera traps. Since 2019, thousands of wildlife biologists have used SpeciesNet through a Google Cloud-based tool called Wildlife Insights to streamline biodiversity monitoring and inform conservation decision-making. The SpeciesNet AI model release will enable tool developers, academics and biodiversity-related startups to scale monitoring of biodiversity in natural areas.
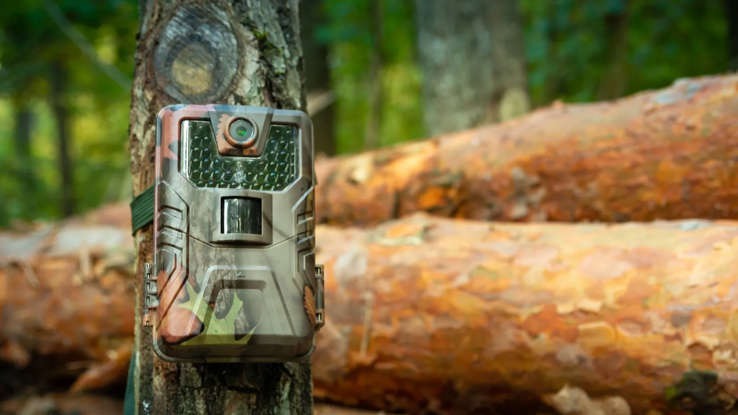
“Werner further emphasized, “Protecting nature and biodiversity is critical to tackling climate change, and we look forward to supporting the startups focused on this challenge.”
similar posts
These are some of the documented impacts:
In one notable case in Brazil’s Pantanal region, researchers used SpeciesNet to analyze over 50,000 camera trap images, identifying critical jaguar habitats and movement patterns. This data enabled conservationists to work with local communities on targeted preservation strategies. Community workshops based on this precise data led to improved understanding of jaguar behavior, significantly reducing human-wildlife conflict incidents by approximately 40% over two years.
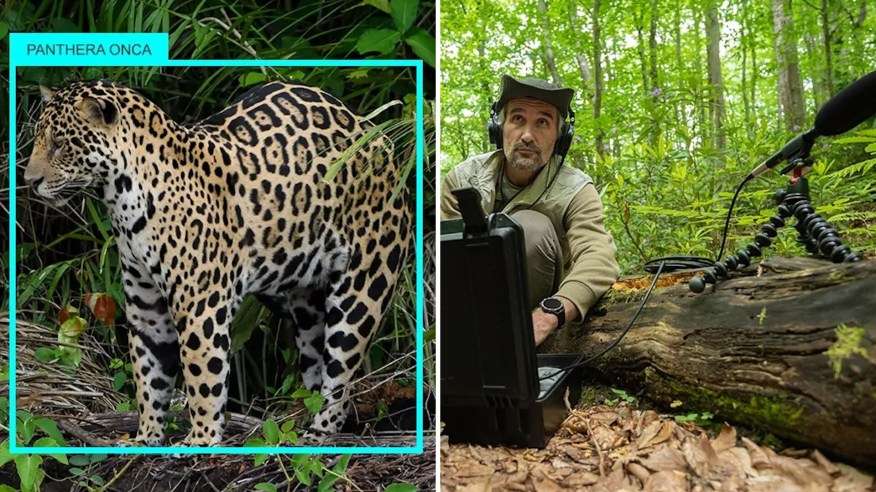
In the Peruvian Amazon, research teams deployed SpeciesNet to analyze which species were utilizing artificial canopy bridges constructed over roads. The AI’s ability to process night-time photos with high accuracy revealed that arboreal mammals were using these bridges 3-4 times more frequently than previously estimated through manual image review.
According to a WWF-Australia conservationist report, image processing speed increased five to tenfold when using SpeciesNet software. This enhanced capability positively influences the response time of conservation teams to threats such as poaching or habitat destruction, often enabling intervention within hours rather than days.
Why Being Open-Source Matters
SpeciesNet is released under the Apache 2.0 license, making it freely available for use, modification, and distribution. This open-source approach is perhaps one of its most significant aspects. As data processing becomes increasingly technology-dependent, conservation organizations can leverage powerful AI without additional staffing costs.
However, challenges in data management remain. While manual data handling can introduce errors, these typically result from hardware failures, software bugs, or human error rather than widespread virus infections as previously suggested.
The open-source nature of SpeciesNet means the entire codebase is accessible to anyone with the technical capabilities to use it. This accessibility enables scientists, developers, and wildlife conservation organizations to customize and enhance the software for specific regional needs. For example, teams in Southeast Asia have already adapted the model to better identify locally endemic species that were underrepresented in the original training data.
This open approach transforms SpeciesNet into a truly global collaboration tool, unrestricted to any single enterprise since it belongs to the worldwide conservation community.
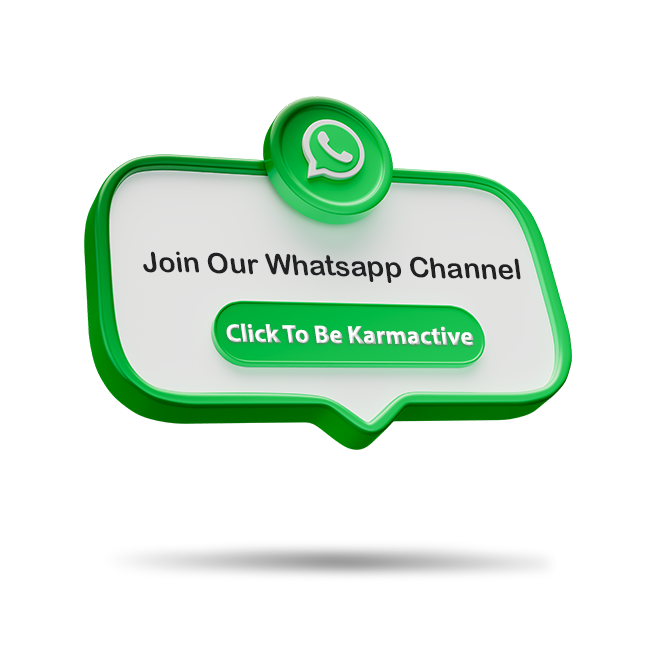
Final Thought,SpeciesNet represents not just an impressive technological achievement but a paradigm shift in conservation methodology—where AI serves not to replace human expertise but to amplify it, accelerating research and protecting wildlife before it’s too late.
Through tools like SpeciesNet, we gain hope for preserving our world’s rich biodiversity. It is with such smart, open, and collaborative technology that we are better able to live in harmony with wildlife and support conservation efforts globally.